일 | 월 | 화 | 수 | 목 | 금 | 토 |
---|---|---|---|---|---|---|
1 | 2 | 3 | 4 | 5 | ||
6 | 7 | 8 | 9 | 10 | 11 | 12 |
13 | 14 | 15 | 16 | 17 | 18 | 19 |
20 | 21 | 22 | 23 | 24 | 25 | 26 |
27 | 28 | 29 | 30 |
- face forgery detection
- neighboring pixel relationships
- f2dnet
- unconditional ddpm
- linear inverse problem
- stochastic contraction theory
- Ai
- conditional diffusion
- object detection
- facenet
- ML
- diffusion
- ssda-yolo
- deepfake detection
- IR
- ilvr
- focal detection network
- ddpm
- pedestrian detection
- unconditional generative models
- image restoration
- ccdf
- hqs-algorithm
- ddim
- Triplet
- semi-supervied learning
- ddrm
- fourmer
- diffpir
- daod
- Today
- Total
목록ddpm (2)
Stand on the shoulders of giants
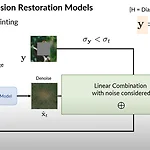
Introduction 본 논문은 Image restoration(IR)에서의 linear inverse problems를 해결하기 위해 제안된 unsupervised posterior sampling 방법론으로, pre-trained denoising diffusion generative model을 활용한다. 학습된 priors에 기반한 unsupervised 접근들의 경우 재학습 없이 새로 주어지는 문제에 adapt할 수 있기에 supervised보다 desirable하다. signal을 복원하기위해 보통의 방법론들은 아래 과정을 따른다.[1] Prior-related terms over the signalNeural network를 통해 distribution 등의 정보 획득degradation..
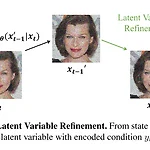
Introduction기존 DDPM은 같은 initial state에서 시작하더라도 비일관된 high-level semantics를 기반으로 stochastic하게 이미지를 생성하기때문에 control하기가 어렵다.Learning-free method이자 generation process에서 각 transition이 reference image를 이용해서 refine되는 모델을 구상하였다. Reference image를 활용해서 coarse ~ fine information 범주로 semantics를 sharing할 수 있도록 하였다. Contributions Generative process의 각 잠재 변수를 주어진 reference image의 것으로 matching함으로써 refine한다.User..